TextFace: Text-to-Style Mapping based Face Generation and Manipulation
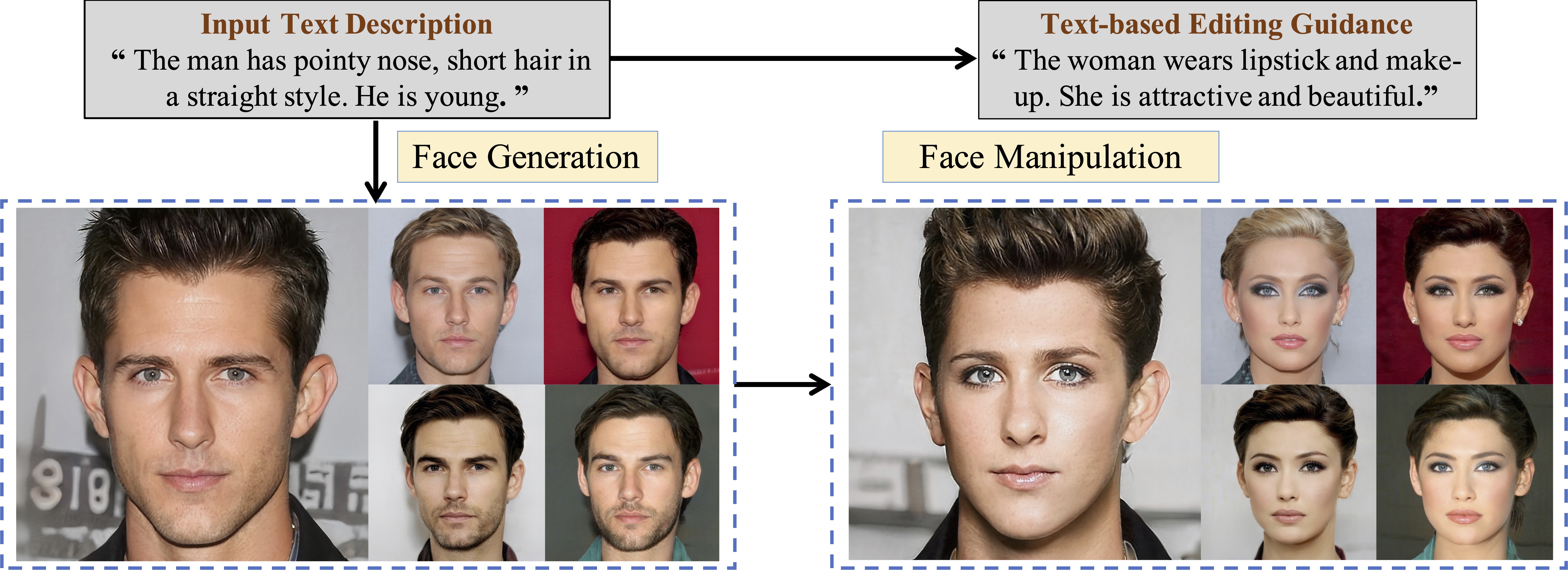
Abstract
As a subtopic of text-to-image synthesis, text-to-face generation has great potential in face-related applications. In this paper, we propose a generic text-to-face framework, namely, TextFace, to achieve diverse and high-quality face image generation from text descriptions. We introduce text-to-style mapping, a novel method where the text description can be directly encoded into the latent space of a pretrained StyleGAN. Guided by our text-image similarity matching and face captioning-based text alignment, the textual latent code can be fed into the generator of a well-trained StyleGAN to produce diverse face images with high resolution (1024 x 1024). Furthermore, our model inherently supports semantic face editing using text descriptions. Finally, experimental results quantitatively and qualitatively demonstrate the superior performance of our model.
Overview
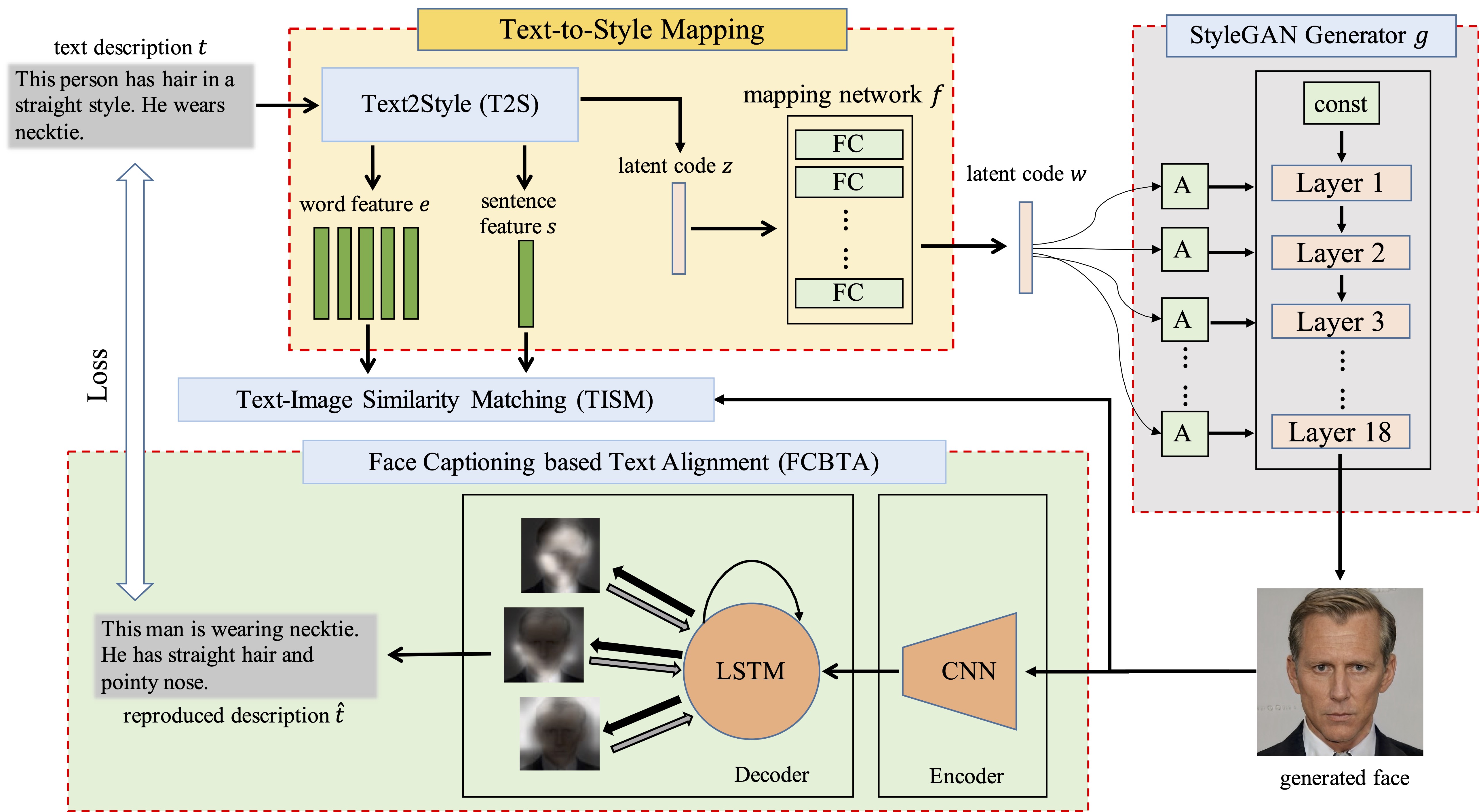
Results
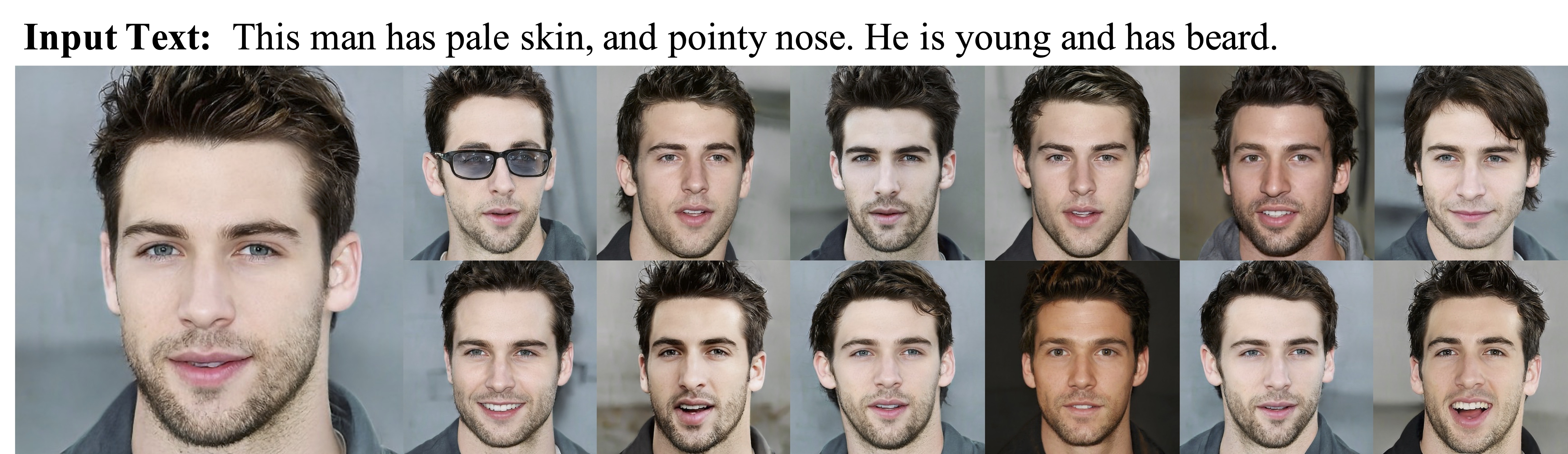
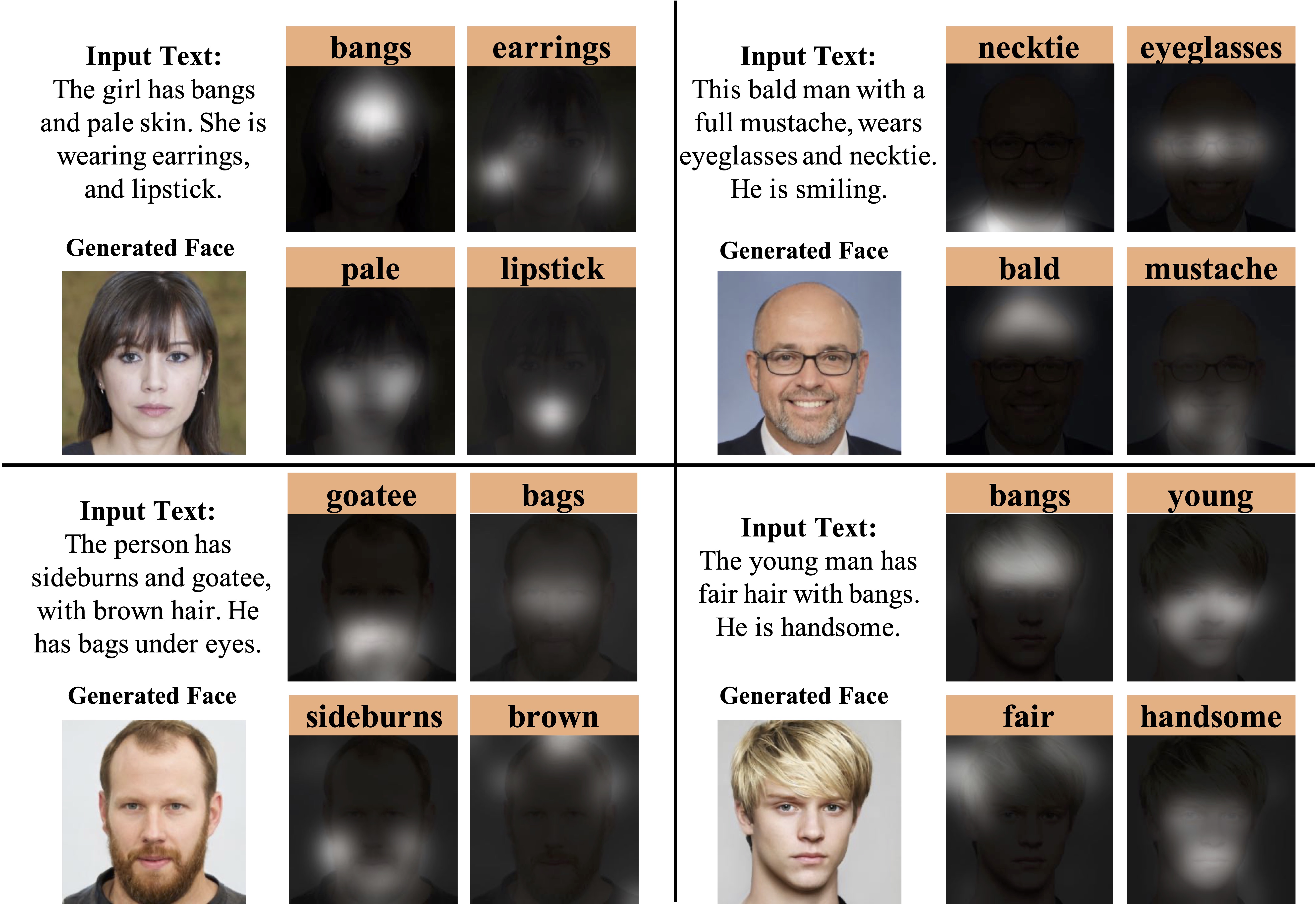
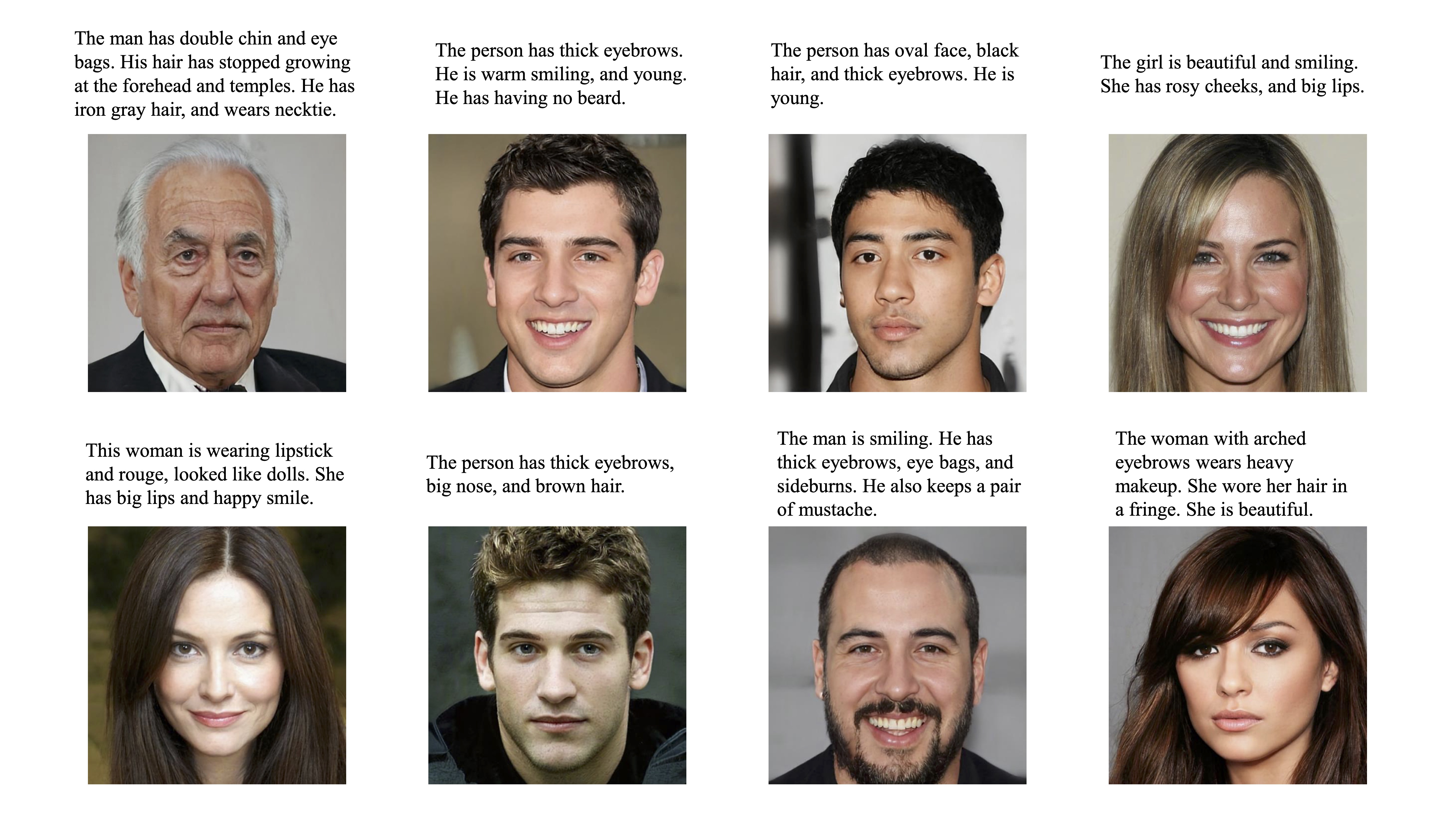
@article{hou2022textface,
title={TextFace: Text-to-Style Mapping based Face Generation and Manipulation},
author={Hou, Xianxu and Zhang, Xiaokang and Li, Yudong and Shen, Linlin},
journal={IEEE Transactions on Multimedia},
year={2022},
publisher={IEEE}
}